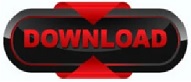
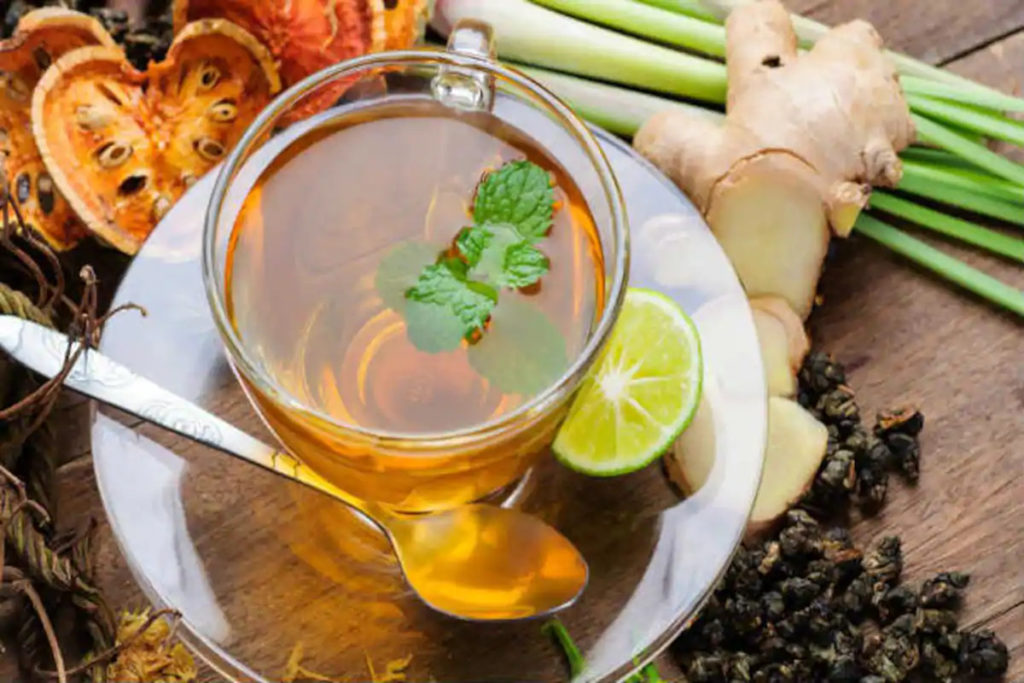
Concerning the type of data, clinical datasets were mainly used. Support vector machines (SVM) arise as the most successful and widely used algorithm.

In general, 85% of those used were characterized by supervised learning approaches and 15% by unsupervised ones, and more specifically, association rules.

A wide range of machine learning algorithms were employed. The aim of the present study is to conduct a systematic review of the applications of machine learning, data mining techniques and tools in the field of diabetes research with respect to a) Prediction and Diagnosis, b) Diabetic Complications, c) Genetic Background and Environment, and e) Health Care and Management with the first category appearing to be the most popular. Extensive research in all aspects of diabetes (diagnosis, etiopathophysiology, therapy, etc.) has led to the generation of huge amounts of data. Diabetes mellitus (DM) is defined as a group of metabolic disorders exerting significant pressure on human health worldwide. To this end, application of machine learning and data mining methods in biosciences is presently, more than ever before, vital and indispensable in efforts to transform intelligently all available information into valuable knowledge. The remarkable advances in biotechnology and health sciences have led to a significant production of data, such as high throughput genetic data and clinical information, generated from large Electronic Health Records (EHRs). Kavakiotis, Ioannis Tsave, Olga Salifoglou, Athanasios Maglaveras, Nicos Vlahavas, Ioannis Chouvarda, Ioanna Machine Learning and Data Mining Methods in Diabetes Research. PERFORMING.341.280.5500 pm I " Aw Sig rill Ia 2110-01 SECTION 1 INTRODUCTION 1.1 BACKGROUND Research in machine learning has taken two directions in the problem of FUNDING NUMBERS MACHINE LEARNING C - F19628-89-C-0001 PE - 62702F PR - MOlE S. To achieve this goal, learning methods were designed, implemented and experimented within several different problem solving environments.ĭTIC i.LE COPY RADC-TR-90-25 Final Technical Report April 1990 MACHINE LEARNING The MITRE Corporation Melissa P. Another major theme is the development of robust machine learning programs that can be integrated with a variety of intelligent systems. In particular, emphasis was placed on representing and using spatial information about three dimensional objects and constraints on the arrangement of these objects in space. The research focused on integrating different representation methods to describe different kinds of knowledge more effectively than any one method can alone. The major goal of the research was to develop flexible, effective methods for representing the qualitative knowledge necessary for solving large problems that require symbolic reasoning as well as numerical computation. Research in knowledge representation, machine learning, and knowledge acquisition performed at Knowledge Systems Lab. Research on knowledge representation, machine learning, and knowledge acquisition
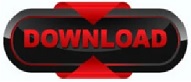